I owe our readers an apology. I clearly wasn’t thinking about election-related burnout when I picked November 11, 2020 to write a follow-up post on the relationship between presidential voting in 2016 and the legacy of slavery in the American South. If you can forgive me, I would like to share some more results from this analysis. In my last post, I showed how you can use network analysis to deal with changing boundaries when working with areal units such as counties and precincts. The basic idea was that if we think about boundary changes as a form of exchange network, we can identify common geographies by identifying the connected components of the resulting graph. We can then aggregate clusters of connected observations to produce observations that are comparable over time, as evidence by our ability to link variation in Democratic vote share in 2016 to variation to the relative size of the enslaved population in 1860.
While aggregation has been used to good effect in a number of high-profile publications (e.g., Bailey and Snedker 2011; Gullickson 2010; Tolnay, Deane, and Beck 1996), it comes at a cost. Think about the Democratic voting example, where we began with 887 counties from 1860 and 1143 counties from 2010 but ended up with only 531 constant counties/county clusters. That’s a lot of information to lose! What if instead of aggregating up, we could project the data from 1860 onto the 2010 boundaries, thus preserving the maximum number observations. If you recall from my last post, the relationship between the source values ys and the target values yt is given by yt = Wys. The trick is to estimate the values making up the nt x ns weights matrix W, which depicts the share of each source value received by each target observation. Perhaps the simplest way of estimating the values making up W is to calculate the proportion of each source observation that overlaps each target.
This approach is known as areal weighting. Within the field of historical sociology, the use of areal weighting goes back at least as far as the work of John Markoff and Gilbert Shapiro, who show how to use estimates of common area and common population to reallocate both absolute figures, as well as proportions. This work was carried as part of a massive data collection project that led not only to innovations in the treatment of spatial data, but to early work on quantitative text analysis, eventually culminating in two large-scale studies of the relationship between revolutionary claim-making in the cahiers de doléances and the fall of the Old Regime in France. Much like the methods considered in my previous post, areal weighting can be understood in network analytic terms. This is evident in the figure below, which depicts the “flow” of land from 1860 counties to 2010 counties, with the magnitude of the flow denoted by the shading of the ties. The darker the tie the larger the overlap between source and target.
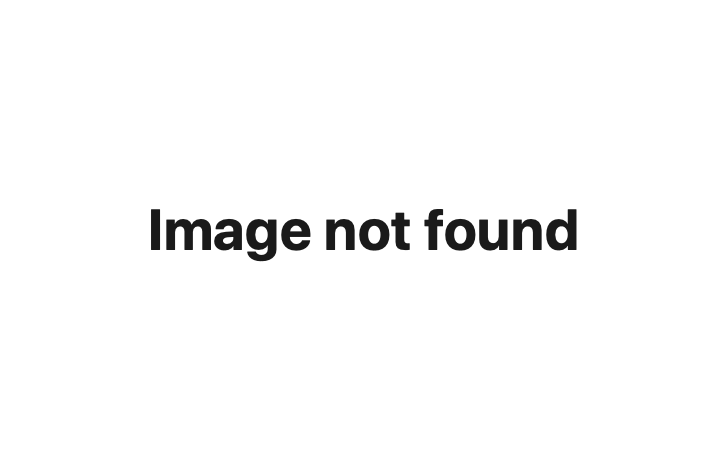
The structure of the graph is a reflection of the mathematical properties of the areal weighting process. This is most apparent in the fact that ties are directed from 1860 counties toward 2010 counties, highlighting the fact that values are being projected from the former onto the latter. The amount that is projected from county to another is a function of the degree of overlap between them. Let’s say, for example, that we are reallocating the 1860 population from Wythe County, Virginia to Bland County, Virginia in 2010. A quarter of Wythe County’s area overlaps with Bland County, which means that a quarter of Wythe County’s 12,305 residents in 1860 will be reallocated to Bland County. An 1860 county can overlap with more than one 2010 county, but it can never give away more than its total area. To put it somewhat more formally, the sum of the edge weights associated with any given 1860 county will always sum to one. This means that a strong tie with one county will necessarily be accompanied by weak ties elsewhere in the system, ensuring that the sum of the estimated values associated with the set target observations is equal to the original sum from which we started. While I initially dropped ties with weights equal to less than half a percent for the purpose of visualization, this turned out to be statistically useful. Once we drop ties, the exchange network breaks into distinct components, the same way that it did when we were identifying common geographies in the previous post. This information can used to help account for the effects of interpolation.
The figure below shows the estimated percentage of the population that was enslaved in 1860 for each of the counties in 2010. When we project values on to the target geography, we are, in effect, estimating the amount of a given variable in a particular area, allowing us to retain more detail. We only know the value for sure when an observation stays the same over time. The chief problem with areal weighting is that it depends on the assumption that values are evenly distributed within target observations, which opens up the possibility of bad estimates. To the extent that areal weighting is organized around the reallocation of values, overestimating the value associated with one observation means that we are necessarily underestimating the value associated with another, and vice versa. As a result, measurement error will tend to propagate within clusters of adjacent observations, giving rise to residual autocorrelation.
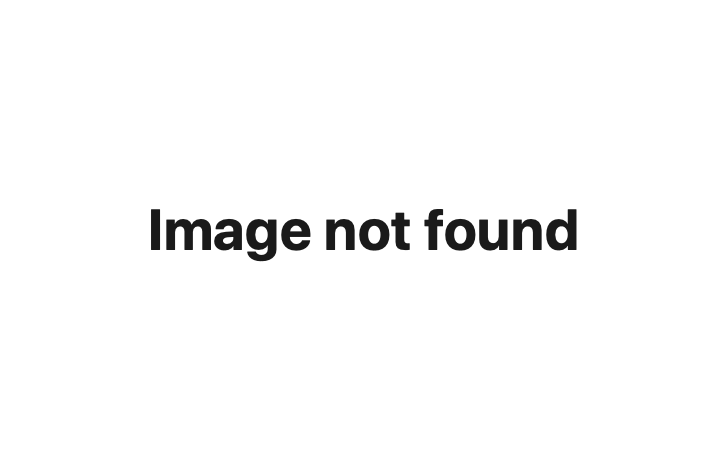
There a number of different ways of correcting for this problem. One possibility is to use clustered standard errors based on the components observed in the geographic exchange network. While this will adjust the standard errors, we will still get the same points that we would get using conventional linear regression, as we did in the previous post. Another possibility is to use maximum likelihood to estimate a spatial error model, which explicitly parametrizes the degree of residual dependence among neighboring observations. The problem in this case is that the outcome is a proportion, which implies heteroskedasticity, thus undermining the consistency of the estimator. We can partially get around this by treating the case-specific variances as draws from a prior distribution. This sounds complicated, but remarkably enough we can get there simply by treating the outcome as if it were t-distributed. As the distribution of estimates below shows, the choice of model has a noticeable impact on the estimated effect of the percentage of the population that was enslaved in 1860 on the size of the Democratic vote share in 1860. While an unadjusted linear regression model produces results that look very similar to what we saw in the last post, the other models look quite different, moving progressively closer to zero with each further refinement.
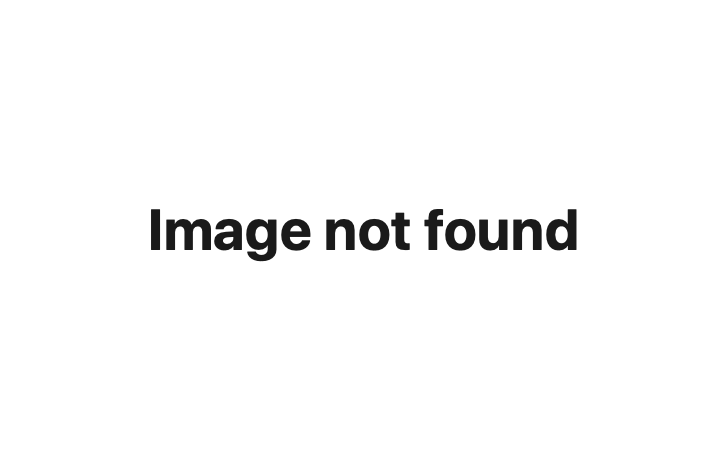
On its face, this may seem overly technical. But I think it is worth pointing out how quickly the practice of quantitative history becomes a game of statistical Whac-a-Mole. We solve the problem of losing observations by using areal weighting, only to introduce the prospect of spatially autocorrelated errors. We solve the problem of spatially autocorrelated errors by using a spatial error model, only to introduce the problem of heteroskedasticity. This is a familiar experience for me, though I’m not sure that I have ever solved every problem in a given analysis. The trick is learning how to take stock and figure which problems absolutely need solving. Spatial autocorrelation may be one of them. In a recent paper, for example, Morgan Kelly argues that existing studies of historical persistence have tended to confuse spatial noise for statistical significance. I would like to come back to this idea in future posts. The great irony is that quantitative historians are often forced to work with spatial data because it is all that is available, yet they often ignore the spatial aspect of the story. There are, of course, also some really cool exceptions.